Note
Click here to download the full example code
Parallel BetweennessΒΆ
Example of parallel implementation of betweenness centrality using the multiprocessing module from Python Standard Library.
The function betweenness centrality accepts a bunch of nodes and computes the contribution of those nodes to the betweenness centrality of the whole network. Here we divide the network in chunks of nodes and we compute their contribution to the betweenness centrality of the whole network.
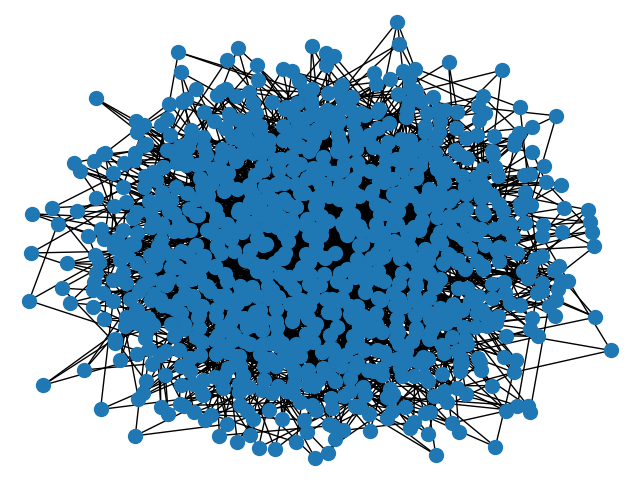
Out:
Computing betweenness centrality for:
Name:
Type: Graph
Number of nodes: 1000
Number of edges: 2991
Average degree: 5.9820
Parallel version
Time: 1.4660 seconds
Betweenness centrality for node 0: 0.04333
Non-Parallel version
Time: 3.9905 seconds
Betweenness centrality for node 0: 0.04333
Computing betweenness centrality for:
Name:
Type: Graph
Number of nodes: 1000
Number of edges: 5109
Average degree: 10.2180
Parallel version
Time: 1.9035 seconds
Betweenness centrality for node 0: 0.00176
Non-Parallel version
Time: 5.0674 seconds
Betweenness centrality for node 0: 0.00176
Computing betweenness centrality for:
Name:
Type: Graph
Number of nodes: 1000
Number of edges: 2000
Average degree: 4.0000
Parallel version
Time: 1.2873 seconds
Betweenness centrality for node 0: 0.00735
Non-Parallel version
Time: 4.3169 seconds
Betweenness centrality for node 0: 0.00735
from multiprocessing import Pool
import time
import itertools
import matplotlib.pyplot as plt
import networkx as nx
def chunks(l, n):
"""Divide a list of nodes `l` in `n` chunks"""
l_c = iter(l)
while 1:
x = tuple(itertools.islice(l_c, n))
if not x:
return
yield x
def betweenness_centrality_parallel(G, processes=None):
"""Parallel betweenness centrality function"""
p = Pool(processes=processes)
node_divisor = len(p._pool) * 4
node_chunks = list(chunks(G.nodes(), int(G.order() / node_divisor)))
num_chunks = len(node_chunks)
bt_sc = p.starmap(
nx.betweenness_centrality_subset,
zip(
[G] * num_chunks,
node_chunks,
[list(G)] * num_chunks,
[True] * num_chunks,
[None] * num_chunks,
),
)
# Reduce the partial solutions
bt_c = bt_sc[0]
for bt in bt_sc[1:]:
for n in bt:
bt_c[n] += bt[n]
return bt_c
G_ba = nx.barabasi_albert_graph(1000, 3)
G_er = nx.gnp_random_graph(1000, 0.01)
G_ws = nx.connected_watts_strogatz_graph(1000, 4, 0.1)
for G in [G_ba, G_er, G_ws]:
print("")
print("Computing betweenness centrality for:")
print(nx.info(G))
print("\tParallel version")
start = time.time()
bt = betweenness_centrality_parallel(G)
print(f"\t\tTime: {(time.time() - start):.4F} seconds")
print(f"\t\tBetweenness centrality for node 0: {bt[0]:.5f}")
print("\tNon-Parallel version")
start = time.time()
bt = nx.betweenness_centrality(G)
print(f"\t\tTime: {(time.time() - start):.4F} seconds")
print(f"\t\tBetweenness centrality for node 0: {bt[0]:.5f}")
print("")
nx.draw(G_ba, node_size=100)
plt.show()
Total running time of the script: ( 0 minutes 29.012 seconds)